Emergence and the Ising model
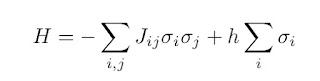
The Ising model is emblematic of “toy models” that have been proposed and studied to understand and describe emergent phenomena. Although originally proposed to describe ferromagnetic phase transitions, variants of it have found application in other areas of physics, and in biology, economics, sociology, neuroscience, complexity theory, … Quanta magazine had a nice article marking the model's centenary. In the general model there is a set of lattice points {i} with a “spin” {sigma_i = +/-1} and a Hamiltonian where h is the strength of an external magnetic field and J_ij is the strength of the interaction between the spins on sites i and j. The simplest models are where the lattice is regular, and the interaction is uniform and only non-zero for nearest-neighbour sites. The Ising model illustrates many key features of emergent phenomena. Given the relative simplicity of the model, exhaustive studies since its proposal in 1920, have given definitive answers to questions often debat